Table of Contents
In the realm of the US stock market, where every second counts and decisions can make or break fortunes, the ability to predict market movements accurately is invaluable. Harnessing data through sophisticated prediction algorithms has become a cornerstone of successful trading strategies. In this comprehensive guide, we delve into the intricate world of US stock market prediction algorithms, exploring how they work, their applications, and their impact on investment decision-making.
Introduction to US Stock Market Prediction Algorithms
US stock market prediction algorithms are sophisticated mathematical models that analyze historical market data to forecast future price movements. These algorithms utilize a variety of techniques, including statistical analysis, machine learning, and artificial intelligence, to identify patterns and trends in market data. By processing vast amounts of information quickly and accurately, these algorithms provide investors with valuable insights into potential market movements, helping them make more informed trading decisions.
Understanding How Prediction Algorithms Work
At the heart of US stock market prediction algorithms lies the concept of data analysis. These algorithms analyze historical market data, such as stock prices, trading volumes, and market indicators, to identify patterns and trends that may indicate future price movements. By applying statistical techniques and machine learning algorithms, these models can identify correlations between different variables and make predictions based on historical patterns. The key to their effectiveness lies in their ability to adapt and learn from new data, allowing them to continuously improve their accuracy over time.
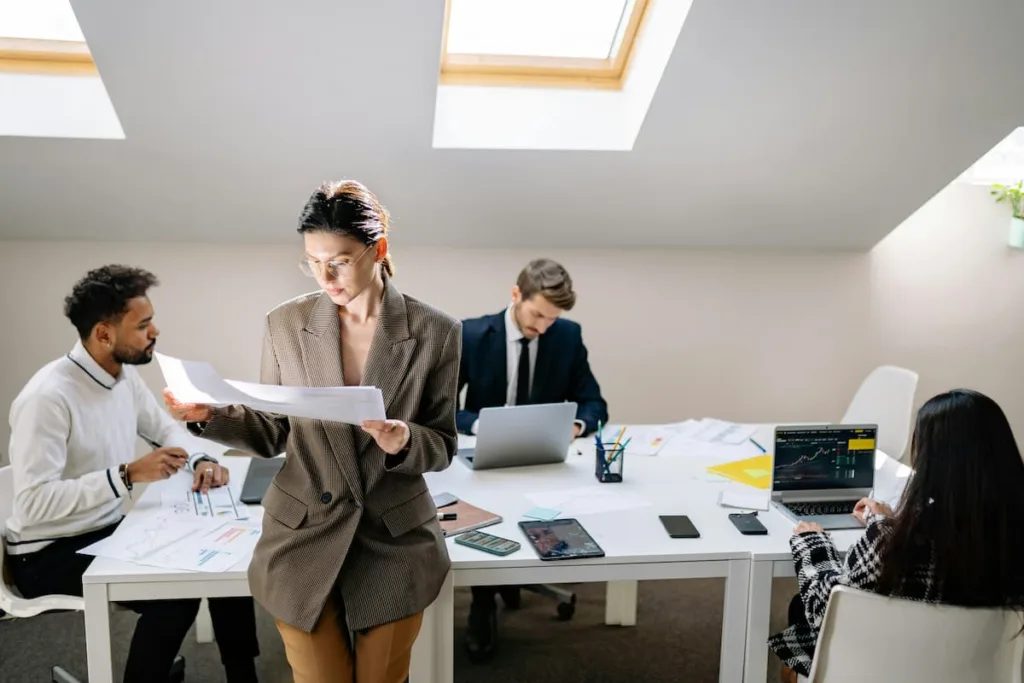
Types of Prediction Algorithms
There are several types of prediction algorithms used in the US stock market, each with its own strengths and weaknesses. Some of the most common types include:
1. Regression Analysis
Regression analysis is a statistical technique used to model the relationship between a dependent variable (such as stock prices) and one or more independent variables (such as market indicators). By fitting a regression model to historical data, analysts can make predictions about future price movements based on changes in the independent variables.
2. Time Series Analysis
Time series analysis is a statistical technique used to analyze sequential data points collected over time. In the context of the stock market, time series analysis can be used to identify trends, seasonality, and other patterns in historical price data. By extrapolating these patterns into the future, analysts can make predictions about future price movements.
3. Machine Learning Algorithms
Machine learning algorithms, such as neural networks and decision trees, are increasingly being used to predict stock market movements. These algorithms can analyze large datasets and identify complex patterns that may not be apparent to human analysts. By continuously learning from new data, machine learning algorithms can adapt to changing market conditions and improve their accuracy over time.
Applications of Prediction Algorithms in the Stock Market
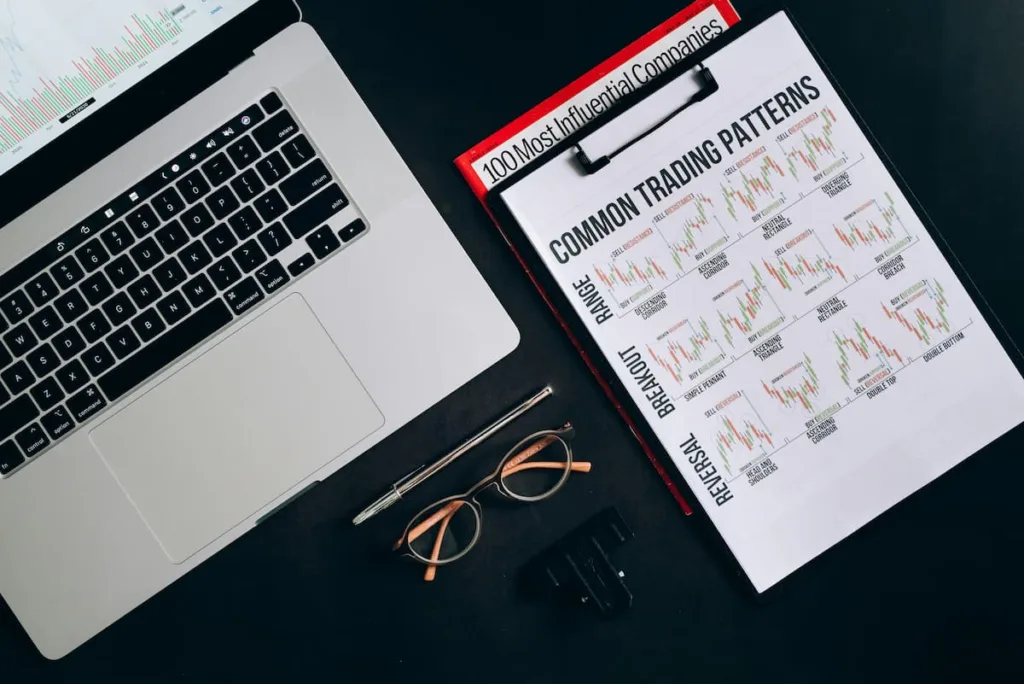
US stock market prediction algorithms have a wide range of applications, including:
1. Trading Strategies
Prediction algorithms are used by traders to develop quantitative trading strategies that capitalize on short-term price movements. By identifying patterns and trends in market data, these algorithms can generate buy and sell signals that help traders make profitable trades.
2. Risk Management
Prediction algorithms are also used by investors to manage risk in their portfolios. By predicting potential market downturns or volatility spikes, these algorithms can help investors adjust their portfolios to minimize losses and preserve capital.
3. Portfolio Optimization
Prediction algorithms can also be used to optimize investment portfolios for maximum returns. By analyzing historical market data and simulating different portfolio allocations, these algorithms can identify the optimal mix of assets that maximizes returns while minimizing risk.
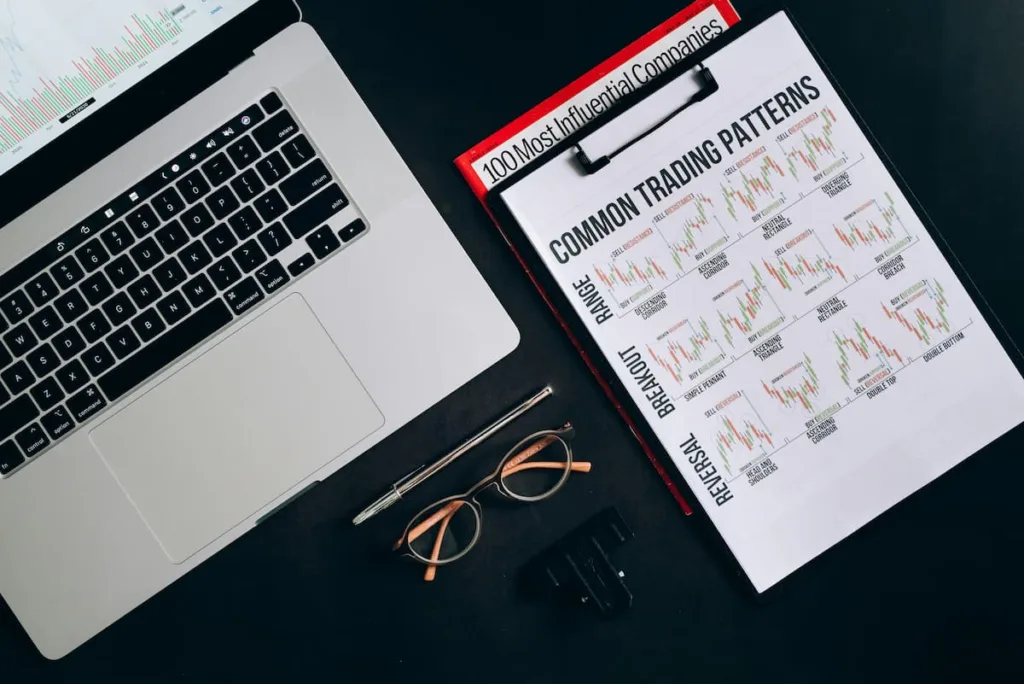
Challenges and Limitations
While US stock market prediction algorithms offer significant benefits, they also face several challenges and limitations. These include:
1. Data Quality
The accuracy of prediction algorithms depends on the quality and reliability of the data used to train them. Poor-quality data can lead to inaccurate predictions and poor investment decisions.
2. Overfitting
Overfitting occurs when a prediction algorithm is trained too closely to historical data, resulting in poor performance on new data. This can lead to misleading predictions and unreliable investment strategies.
3. Market Volatility
Prediction algorithms may struggle to accurately forecast market movements during periods of high volatility or uncertainty. Sudden changes in market conditions can confound the algorithms and lead to inaccurate predictions.
Conclusion
In conclusion, US stock market prediction algorithms play a crucial role in today’s investment landscape, providing investors with valuable insights into market trends and helping them make more informed trading decisions. By harnessing the power of data analysis, statistical techniques, and machine learning, these algorithms offer a powerful tool for navigating the complexities of the stock market. While they face challenges and limitations, their potential to enhance investment performance and manage risk makes them an indispensable asset for investors.
Please click here for Further Exploration
Please click here to read about STAY AHEAD OF THE CURVE: ADVANCED US STOCK MARKET PREDICTIVE METHODS
1 thought on “Harnessing Powerful Data: US Stock Market Prediction Algorithms”